Imagine the only way to complete an assignment was to sort and sift through a towering pile of papers—reading each one—to retrieve a critical piece of information by the end of your workday. After hours of sorting, you finally get through that stack of paper. Success, right? Only for a moment. Tomorrow, another critical piece of information is demanded just as another stack appears on your desk—and so the process begins again. And again the next day. And the next.
For data scientists, this isn’t a hypothetical. This is the job—albeit with troves of digital information instead of paper.
Advancements in AI, specifically within machine learning, improve the efficiency of this process, pulling out the microscopic and even macroscopic data required. Most of the data collected will be insignificant—normal data that can be compressed and stored until needed – while some will indicate onset threats—actionable, smarter data. Without smart data and compute technology, some data-crunching tasks could take weeks or even months to complete. The overwhelming inundation of data is both the enemy and the solution for data scientists.
And big data is what you deal with when you look to solve big problems such as the global food supply.
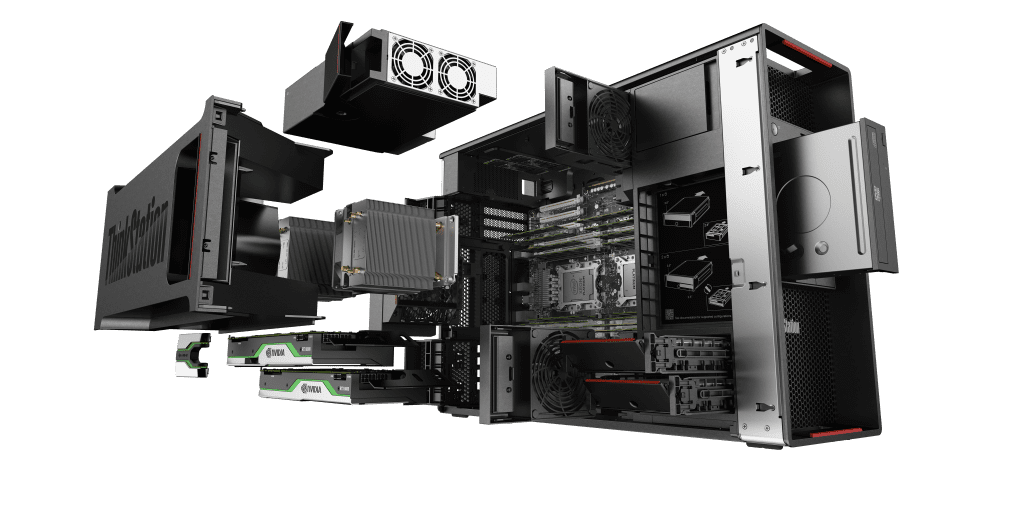
Increasing population and urbanization are leading to a shortage of food, water, energy and arable lands. As a result, it is imperative to study and understand the challenges ahead. This is precisely what the North Carolina State University Geospatial Analytics Researchers have been working on for the past couple of years. Using smart data and artificial intelligence, NCSU researchers preemptively identify agricultural areas and crops that will be affected by climate change, such as floods or droughts.
As farmland disappears and the food demand increases, these researchers are using smarter, data-driven digital agricultural solutions to increase crop production and optimize water and energy utilization. Farmers require actionable knowledge in-hand as soon as possible to ensure the best quality food makes it from the farm to the table.
Space agencies such as NASA are collecting tens of terabytes of data from Earth observing satellites, in addition to petabytes of climate simulations and streaming observations from billions of distributed sensors. Centralizing data from these diverse streams and doing traditional offline analytics alone aren’t meeting the challenges faced by farmers on a daily basis. Extracting critical knowledge, if done efficiently and in near real-time, can result in better harvests and better conservation and less disruption to food production worldwide under fluctuating environmental conditions.
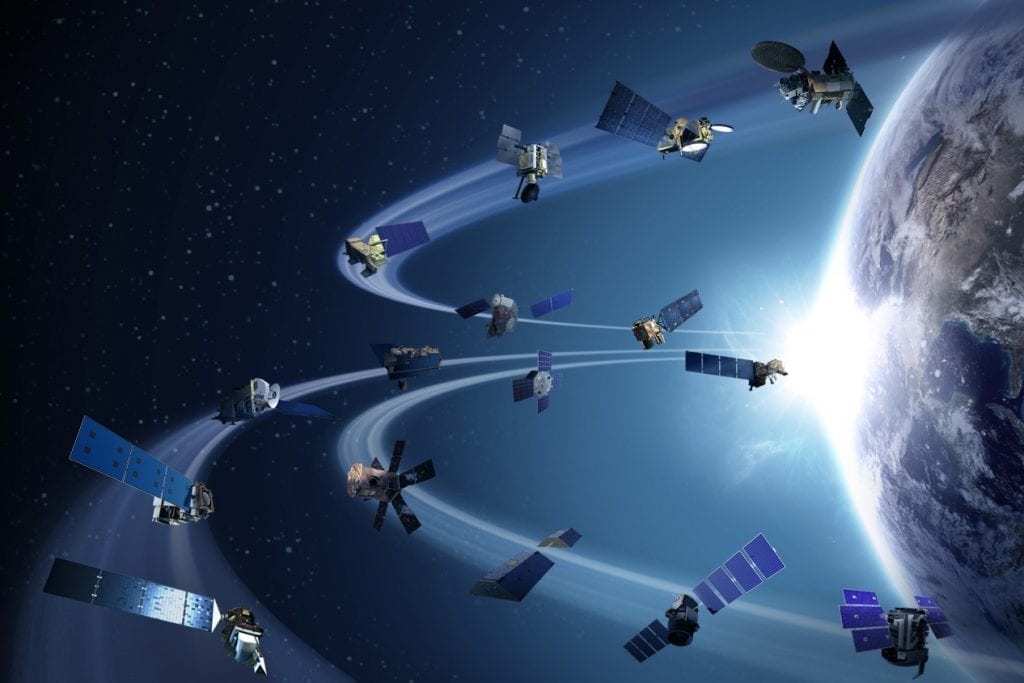
Deluged with data, the team needed both a real-time and an offline analytics solution with enough computing power to provide instant feedback and to compress and store information for broader analysis. The team at NC State turned to Lenovo to help navigate this challenge.
“Success of AI systems, in particular deep learning can be attributed to the availability of big data, however the bottleneck for many organization is the outdated computing infrastructure,” said NC State’s Ranga Raju Vatsavai. “Modern powerful workstations, such as the ThinkStation P920 equipped with the latest Intel Xeon processors and high end GPUs coupled with Lenovo’s LiCO AI framework, are enabling researchers to develop and deploy AI solutions at an unprecedented speed.”
The team needed a two-prong approach to understand what is happening now and to better predict the future. The ThinkStation P920 AI workstation acts as a sandbox, operating in the background, sifting through incoming offline data and learning and updating models from the global data it receives. The ThinkStation P330 Tiny operates as an Edge device collecting data from the sensors in the field, reporting real-time updates and collecting data to be fed back around again. This continuous loop of understanding and compiling offline and real-time analytics results in smarter data—and actionable solutions for NC State to predict crop conditions, in even the most difficult conditions.
Want to get into the weeds on what that data optimization means? Here’s the technical detail:
NC State researchers successfully trained well-known VGG-16 deep learning model for crop classification using very high resolution satellite images collected over North Carolina State. Raw satellite image data accounted for 6 terabytes, and training data accounted for 91 MB. VGG-16 model was trained on a P920 ThinkStation using Intel Xeon Gold 6134 CPU and Lenovo’s LiCO 5.2.1 framework. Unlike traditional CPUs, modern Intel Xeon processors offers additional instruction sets similar to GPUs, which allows optimization of certain computational tasks. Without using such new instruction set optimizations VGG-16 model took approximately 15 hours, whereas the optimized model took only 9 hour 19 minutes, giving significant improvement in per node performance.
AI and machine learning offer accelerated data interpretation with more efficiency and control. Powered by these workstations, agriculture researchers are equipped with the artificial intelligence to work smarter, confident in smart data and solutions to address threats to food security.