Picture this: You’re scrolling through your favorite news app with one hand and holding your favorite cup of coffee in the other; your self-driving car, powered by artificial intelligence (AI), navigates to work while you relax. But it happens to be a foggy morning, casting everything in a gray haze as your car approaches a simple stop sign. The sign is further obscured by dangling tree branches, and a fresh coat of graffiti has transformed the letters STOP to SIOB. Your autonomous car barrels at full speed past the sign and through the intersection, swerving violently to avoid a pedestrian—your coffee spilling onto your lap—before continuing on to your office as if nothing happened. The car failed to properly recognize the stop sign, all because the underlying artificial intelligence had not learned enough.
When many of us read or hear the phrase “artificial intelligence” our minds might jump to advanced robotics, humanoid droids, or the voice that serves as a virtual assistant on our mobile devices. AI has been used to describe a large variety of technological tools and advancements, and oftentimes is reduced to a buzzword and employed as a marketing technique. But what exactly is AI? What defines this frontier and makes it so revolutionary?
“AI is the ability for machines to mimic the cognitive functions and decision making of humans with limited human intervention,” says Robert Daigle, Artificial Intelligence Innovation & Business Leader at Lenovo.
A seemingly autonomous choice—for example, a self-driving car slowing down and stopping when it approaches a stop sign is not truly autonomous, at least not in the same way humans are. The self-driving car is doing two things: first, recognizing the stop sign, and second, following the rule programmed by its creators to stop. That programmed “rule” heavily qualifies the idea of autonomy. But the real “magic” happens in the first step. For the car to recognize the stop sign, a complex process called machine learning unfolds behind the scenes.

Technologies with AI operate under the powerful mechanisms of machine learning and deep learning, where the latter is a subset of the former. Remember the car recognizing the stop sign? First, it must learn what a stop sign is. That’s where machine learning develops a model to pick up on the defining features of a stop sign. The creators of the autonomous car might, for example, program it to scan for octagonal red shapes a few feet off the ground on the right-hand side of the road. It starts simply enough, but real-world complications quickly arise. Imagine graffiti scrawled over the words, or tree branches obscuring the full shape—the car must become smart enough to account for those near-infinite variables. Through trial and error, the machine learning algorithm evolves and improves to better recognize, and this part of the process is designed to happen without much human intervention.
“Once the model is performing well,” says Robert, “it’s deployed into an application or system to make decisions on new data – this is known as inferencing.”
The newer data it encounters and correctly interprets, the more effective the model becomes. Now, in deep learning, the process of inferencing begins earlier, during the training of the algorithm.
Deep learning operates on the same basic principles of machine learning, but instead of programming the technology to look out for specific features, the machine infers the features of something after encountering a large set of examples. For instance, a deep learning algorithm intended to teach the machine to recognize photos of cats might be given millions of images of cats. From these images, it will learn what a cat looks like. But the strength and variety of that source data is essential. For a deep learning algorithm to work as intended, it requires a vast and varied set of data properly labeled as the specific object or situation. If a few photographs of dogs are mistakenly labeled as “cat” and fed into the algorithm, the machine might now mistake pictures of dogs for cats. And that ignores the variables of wild cats, domesticated breeds, image quality, artificial vs. real cats, etc. This sort of mistake can be particularly dangerous when lives are at stake, such as with self-driving vehicles or cancer-recognizing technologies. Even though the machine can learn by itself to identify a cat, humans are still responsible for giving it the pool of information from which to learn.
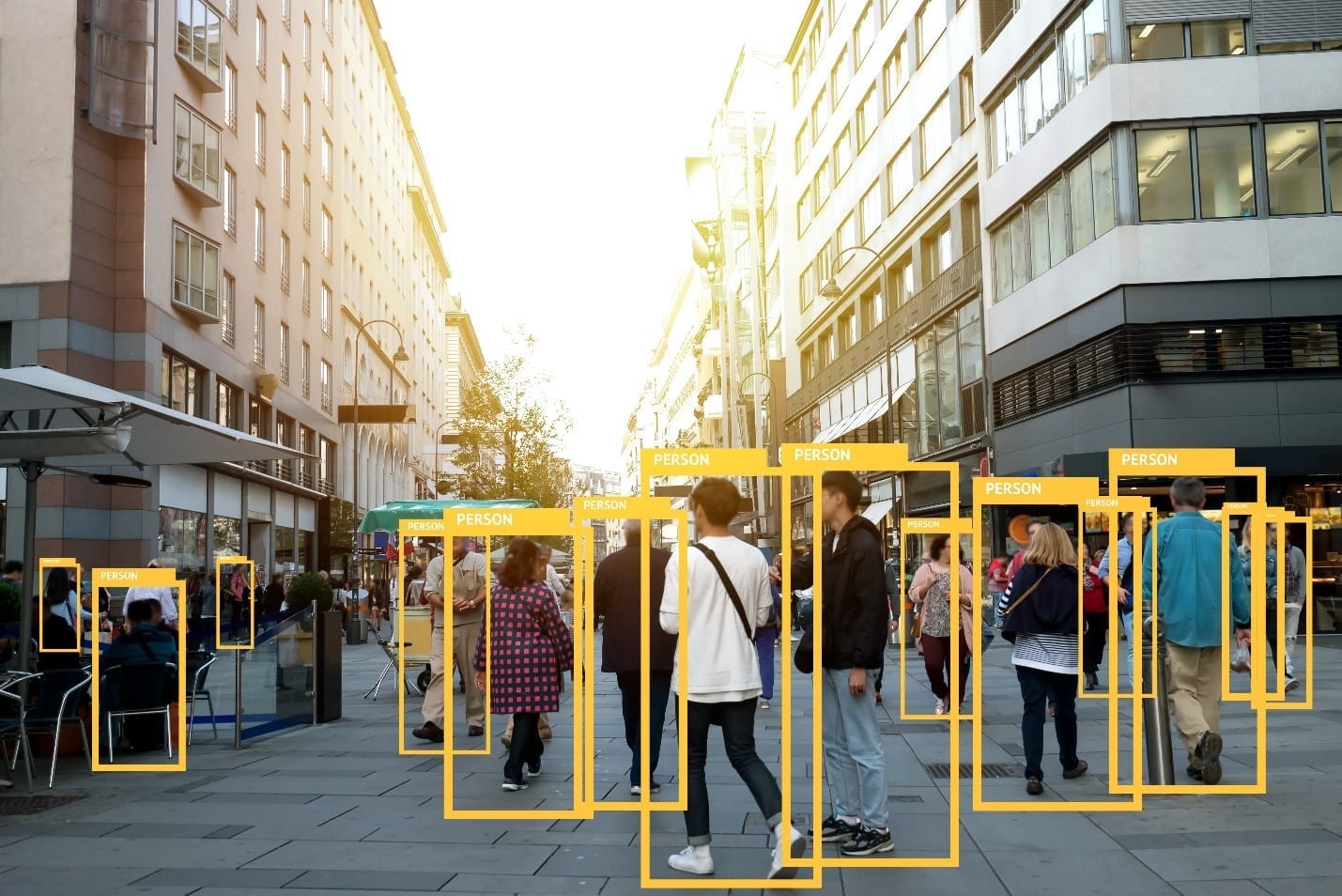
In addition, because the machine is teaching itself, we often do not understand the specific features a deep learning algorithm recognizes to evaluate the nature of an object or a situation.
Howard Locker, Technology Consultant for Lenovo, puts it simply: “No one knows why or how the program knows if a cat is a cat or not. It’s a ‘black box.’”
This can be become an issue in scenarios where the algorithm fails to properly recognize something—for instance, a stop sign. A large part of current AI research explores unpacking deep learning processes to understand why machines make certain decisions. Was it the fog, the branches, the graffiti, or some combination of all three that caused the car to make a mistake? Answering questions like these is vital to improving AI, and because safety is of the utmost importance, we might still be many years away from seeing fleets of self-driving vehicles on the road.
Machine and deep learning can improve lives and increase efficiency in countless ways across industries. Healthcare, manufacturing, home assistance technologies, financial services, and life sciences are just a few of the leading areas where AI is being applied to advance and enhance operations. AI can even have applications in saving lives, for example, in earlier and more accurate detections of cancer, or in recognizing those most in need of immediate emergency assistance. With how quickly artificial intelligence is evolving and being applied to an increasing variety of purposes, there really seems to be no boundaries to which AI can change the world for the better.
At the end of the day, there is no “one size fits all” definition of AI, though machine learning algorithms sit at the heart of the technology. But to define AI with just a single word, Robert, Howard, and Mounika all agree: “Revolutionary.”